- What exactly is artificial intelligence?
- How do algorithms work?
- What is the purpose of artificial intelligence?
- Different types of AI explained
- The various sub-domains of artificial intelligence
- How does artificial intelligence benefit us?
- Ethical considerations and dangers
Although artificial intelligence is already part of almost every aspect of our daily lives, many people – including numerous business leaders – are still not very familiar with the concept of AI, how exactly it can transform their lives and their businesses, and how it affects our economy, governance, and society as a whole.
What exactly is artificial intelligence?
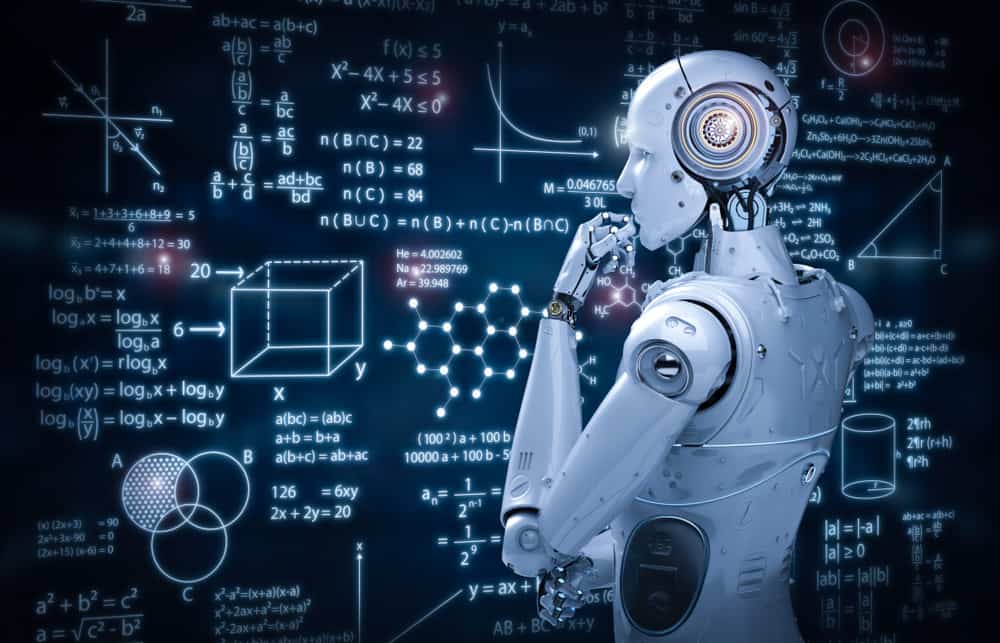
Artificial intelligence is the simulation of natural intelligence in machines that are programmed to learn and mimic the thought patterns of humans. Artificially intelligent machines can be taught to learn from different experiences and perform human-like tasks. In simple terms, artificial intelligence combines computer science with robust datasets which enable us to use the technology for solving all kinds of problems. AI operates in an intelligent and intentional manner and is capable of making decisions that traditionally needed a human level of expertise. It also encompasses the sub-fields of machine learning and deep learning, which use algorithms seeking to create expert systems that make predictions or classifications based on input data.
How do algorithms work?
To put it simply, an algorithm is a set of step-by-step instructions to solve a problem. Computer algorithms work via input and output. They take the input and apply each step of the algorithm to that information to generate an output. Artificial intelligence algorithms are designed to make decisions that are usually based on real-time data. Using sensors, digital data, or remote inputs, algorithms combine information from various sources, instantly analyse the material, and act on the insights derived from this data. Algorithms are designed by humans with intentionality and reach conclusions based on their instant analysis. As a result of massive improvements in storage capacity, processing speeds, and analytic techniques, today’s algorithms are capable of incredible sophistication in analysis and decision making.
Machine learning technology looks for underlying trends in the data it crunches. If it spots something that is relevant for a practical problem, software designers can combine this knowledge with data analytics to gain understanding of specific issues. Big data analytics is the use of advanced analytical techniques against very large, diverse data sets that include structured, semi-structured, and unstructured data from different sources and in different sizes – from terabytes to zettabytes.
Big data is a term to describe data sets whose size or type cannot be captured, managed, and processed by traditional relational databases with low latency. Characteristics of big data include high volume, high velocity, and high variety. For example, the different types of data originate from sensors, devices, video/audio, networks, log files, transactional applications, web, and social media – much of it generated in real time and on a very large scale. Big data analytics enables more efficient and faster decision-making, as well as modelling and predicting of future outcomes and enhanced business intelligence.
AI systems using big data are able to learn and adapt as they compile and crunch information and make decisions. For artificial intelligence to remain effective, it needs to be able to adjust to changing circumstances and conditions, such as environmental factors, financial situations, road conditions, or military circumstances. It does this by integrating these changes in its algorithms and then making decisions on how to adapt to the new circumstances.
What is the purpose of artificial intelligence?
Artificial intelligence simplifies many of our day-to-day tasks, enhances human capabilities, and helps us make advanced decisions fast, based on the best possible background data. If we look at it from a philosophical perspective, artificial intelligence has the potential to help humans live more meaningful lives devoid of hard labour, and helps manage the complex web of interconnected individuals, companies, states, and nations to function in such a way that it is beneficial to all of humanity. Artificial Intelligence has also been touted as our ‘final invention’, a creation that will invent ground-breaking tools and services that will fundamentally transform how we lead our lives by removing strife, inequality, and human suffering. This all sounds very promising, but we are still a long way from those kinds of outcomes.
Artificial intelligence underpins many aspects of modern life, from search engines to banking, and advances in image recognition and machine translation are among the key developments in recent years. In business, the technology is used to improve corporate process efficiencies, automate resource-heavy tasks, and to make business predictions based on hard data instead of gut feelings.
Other, broader uses of AI include:
- Searching within data and optimising the search to give the most relevant results
- If-then reasoning – which can be applied to execute a string of commands based on specific parameters
- Pattern detection to identify significant patterns in large data sets in order to generate unique insights
- Applied probabilistic models for predicting future outcomes
Google’s predictive search algorithm, for instance, uses past user data to predict what you will type next in the search bar to optimise search results. Netflix uses past user data to recommend which movie you might want to see next – with the goal to increase watch time. And Facebook uses past user data and facial recognition technology to automatically suggest which friends to tag in your photos, based on their facial features.
Different types of AI explained
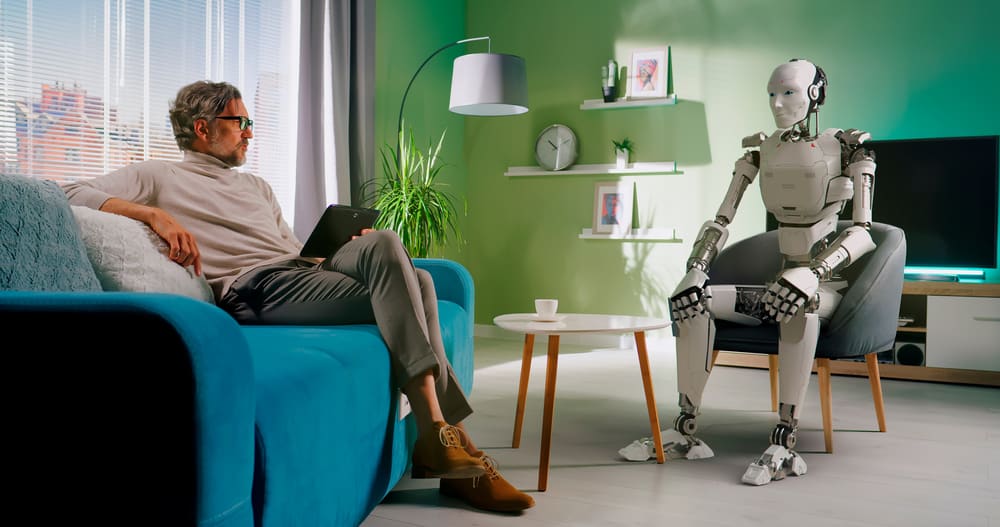
While all artificial intelligence is commonly referred to as AI, there are actually three different types of AI: artificial narrow intelligence (ANI), artificial general intelligence (AGI), and artificial super intelligence (ASI). So, what are the differences between each of these three types of AI?
Artificial narrow intelligence (ANI): performing a single task extremely well
This is the only type of AI that is currently in use, and includes applications like Siri and natural language processing. ANI excels in performing singular tasks by replicating human intelligence, such as the AI that is used in voice assistants and speech recognition systems. This AI closely resembles human functioning in very specific contexts, and in some cases even surpasses them, but only with a limited set of parameters and in very controlled environments, such as when playing chess.
Artificial general intelligence (AGI): performing intellectual tasks the way humans do
AGI would be on the level of a human mind. It is still a theoretical concept, as it would need to comprise thousands of ANI systems working in tandem, communicating with each other to mimic human reasoning. This type of AI would function more comprehensively and could be applied to diverse tasks. It would be able to improve itself by learning and, in terms of its capabilities, would be the closest to the human brain.
Artificial super intelligence (ASI): surpassing human intelligence
ASI would match and then surpass the human mind. This AI concept is way more sophisticated than any other artificial intelligence system or even a human brain. ASI would be able to contemplate abstractions that humans would be unable to comprehend. Its neural network would exceed that of humans – which consists of billions of neurons. Artificial super intelligence would rapidly be able to improve its capabilities and advance into realms that we can’t even fathom today. Not only could this type of AI carry out any conceivable task, but it might even be capable of having emotions and relationships.
The various sub-domains of artificial intelligence
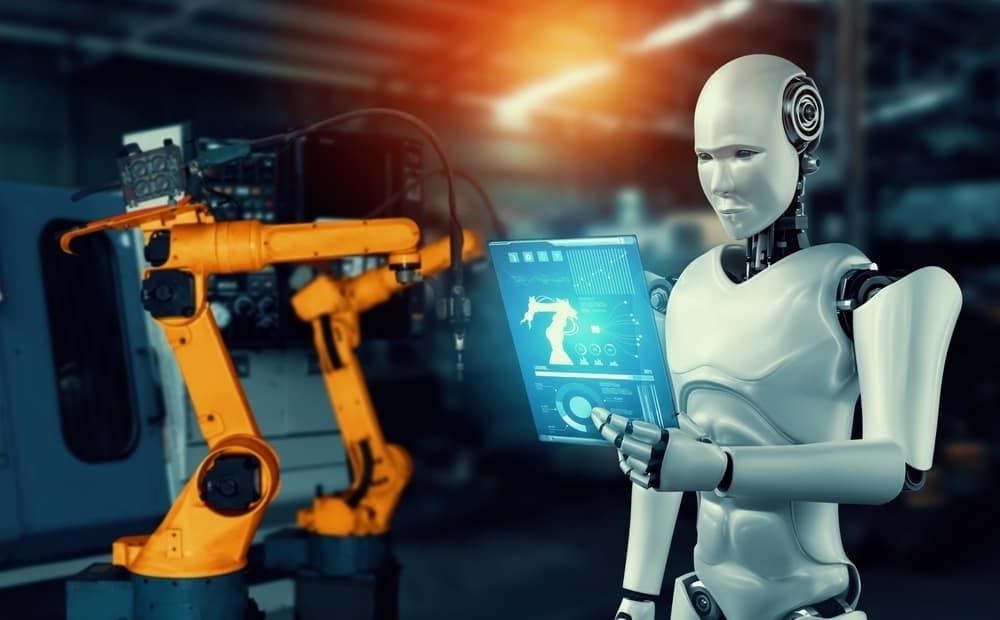
To understand how artificial intelligence actually works and get a feel for the myriad of ways in which it could be applied – and in which fields and industries – one needs to take a deep dive into the various sub-domains of AI.
Machine learning
Machine learning teaches a machine how to make inferences and decisions based on past experiences. It identifies patterns and analyses past data to work out the meaning of these data points and reach a possible conclusion without having to involve human experience. The automated reaching of conclusions by evaluating data saves businesses time and helps them arrive at decisions more efficiently and accurately. Machine learning is the process that powers many of the services we use today, such as the Netflix, Spotify, and YouTube recommendation systems, search engines like Google and Baidu, social-media feeds like Facebook and Twitter, and voice assistants like Siri and Alexa.
Deep learning
Deep learning is used to teach machines what comes naturally to humans. It can be thought of as a way to automate predictive analytics. By continually analysing data with a given logical structure, deep learning algorithms attempt to draw conclusions as humans would. To achieve this, deep learning uses a multi-layered structure of algorithms called neural networks. Unlike machine learning, deep learning doesn’t require human intervention to process data, which enables us to scale machine learning in more interesting ways. For example, deep learning algorithms can automatically translate between languages, which is useful in many different scenarios, such as for travelling, business dealings, and in government processes and decision making.
Neural networks
Neural networks work similar to human neural cells. They are basically a series of algorithms that capture the relationship between various underlying variables and process the data much like a human brain does. From forecasting to market research, they are extensively used in areas like sales and stock exchange prediction, fraud detection, and risk analysis. Neural networks make use of training data to learn and improve their accuracy over time. And once these learning algorithms are fine-tuned for accuracy, they can be extremely powerful tools in artificial intelligence and computer science, and enable us to rapidly classify and cluster data. Tasks in speech or image recognition, which can take humans hours to do, can be completed by neural networks within minutes. One of the most well-known examples of a neural network is Google’s search algorithm.
Natural language processing
NLP is the science of reading, understanding, and interpreting a language by a machine. Once a machine understands what the user intends to communicate, it can respond accordingly. Most NLP techniques use machine learning to draw insights from human language. A common example of NLP is spam detection, in which a computer algorithm is able to determine whether an email is spam. It does this by analysing the subject line or the actual content of an email. Some common applications of NLP include speech recognition, text translation, and sentiment analysis. For example, Amazon uses NLP to help interpret customer reviews and improve client experience, and Twitter uses NLP to scan tweets for terrorism-related language. Many mobile devices incorporate speech recognition technology which enables them to conduct voice search, such as Siri, or to enable autocorrect to make texting more efficient. Other examples include chatbots on e-commerce sites, messaging apps like Facebook Messenger and Slack, and tasks done via voice assistants or virtual assistants.
Computer vision
Computer vision is a field of artificial intelligence that trains computers to interpret and understand the visual world. To make sense of a digital image from a camera or a video file, computer vision algorithms break it down and study all the different components in the image. This way, the machine is able to learn about and classify images and ‘react to what they see’. Whether or not we are consciously aware of it, we use computer vision technology every day. It’s in the facial recognition technology that is used for security and surveillance, shops use it to monitor customers and keep track of their inventories, and self-driving cars use it to recognise different objects on the road. But it is also increasingly used to help prevent fraud or diagnose diseases.
Cognitive computing
Cognitive computing systems already impact every aspect of our lives, from sports and entertainment to travel, fitness, and health. A cognitive computing system learns based on interactions and outcomes instead of simply regurgitating information. In terms of speed and accuracy, cognitive computing systems already rival a human’s ability to answer questions in natural language. It navigates the complexities of natural language and processes and analyses massive amounts of data with incredible speed. It can sort through vast quantities of structured and unstructured data to provide personalised and specific recommendations backed by solid evidence. In healthcare, cognitive systems like IBM Watson collect and analyse data from previous medical reports, medical journals, diagnostic tools, and historical data from the medical fraternity to enable physicians to provide data-backed treatment options.
How does artificial intelligence benefit us?
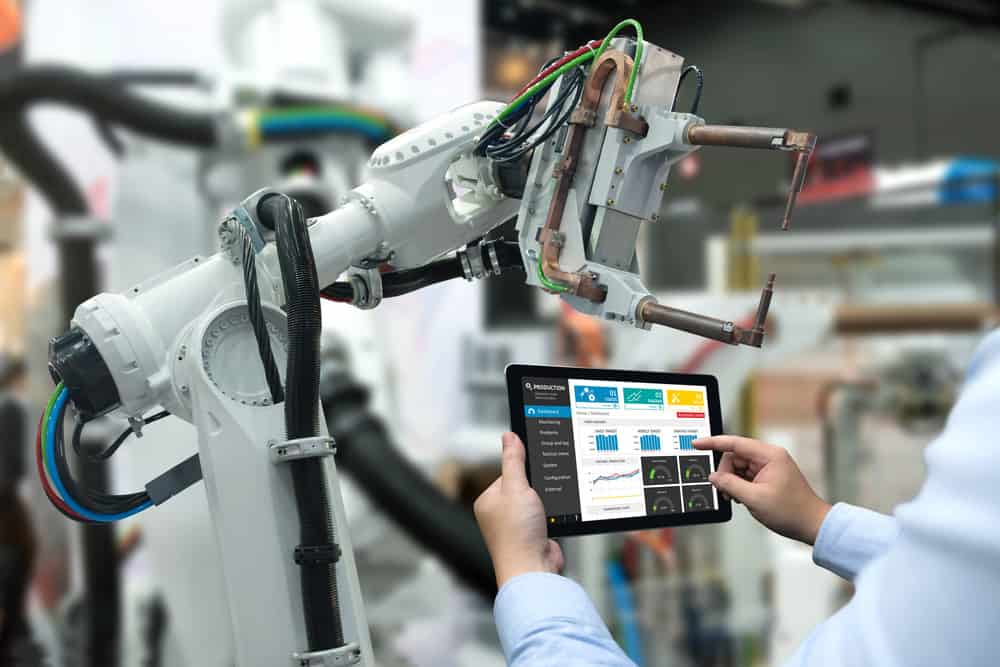
Artificial intelligence has become an integral part of our everyday life. From our smartphones and GPS navigation to mobile banking, fraud prevention, and movie and music recommendations – we can hardly imagine our lives without it. Let’s have a look at some of the many advantages of artificial intelligence.
Increased efficiency
One of the greatest advantages of AI systems is that they enable humans to be more efficient. AI can be leveraged to perform small, repetitive tasks faster, or it can be used to complete much larger, more complex tasks with relative ease. No matter their application, AI systems are unbound by human limitations and can keep going indefinitely. AI is often used to perform the mundane, monotonous, time-consuming tasks that humans wouldn’t find particularly enjoyable. For instance, insurance companies use AI to process claims faster and at higher volumes than a human could, freeing up time for humans to focus on more important matters.
Lower error rates
The human brain can only focus on one task for so long before that focus starts to wane. When we get tired, we’re more likely to make poor decisions and mistakes. Repetitive jobs are particularly prone to human error – when a task is repetitive, it’s easier for humans to lose concentration. But AI systems don’t have to focus. They’re programmed to keep working until we decide they can stop. AI systems greatly reduce the risk of human error and consistently produce extremely accurate results.
24/7 availability
According to the US Bureau of Labour Statistics, Americans work an average of 8.8 hours a day. Whether they’re productive that whole time, however, is an entirely different story. Machines don’t step away from their desks to take coffee or smoke breaks or to catch up with colleagues. And they definitely don’t pack up and go home at 5 pm sharp. Digital assistance solutions like chatbots, for instance, are available to deal with customer inquiries no matter the time of day – or night.
Personalised experiences
Technologies like recommendation engines enable changing recommendations based on client preference, which can be used to create a highly personalised user experience – an approach many companies are taking. Personalised user experiences are a sure-fire way to make anyone feel special. For platforms that wish to improve client engagement, recommendation algorithms can be tweaked to increase engagement by suggesting specific content.
Deeper data analysis
Modern businesses are swimming in data, but are they getting the most out of it? While manual data analysis can be an extremely time consuming exercise, AI systems can process and analyse massive amounts of data at incredible speed. AI systems can quickly find relevant information, identify trends, make decisions, and offer recommendations based on historical data. For instance, algorithms can quickly analyse the effectiveness of marketing materials, identify customer preferences, and offer actionable insights based on those customer behaviours.
Less risk
Artificial intelligence concepts, especially cognitive computing, involve advanced technology that can address complex situations characterised by uncertainty and ambiguity. AI, in combination with traditional analytics and human thought processes, is increasingly used to help augment business decisions and enhance performance. And as risk generally encompasses ambiguous and unlikely events and situations, the domain of risk management is particularly well-suited to cognitive computing capabilities. As cognitive capabilities can greatly enhance traditional analytics to help pinpoint indicators of risk, more and more organisations use big data to be able to act more preventatively.
Less bias in decision making
While human bias can never be completely eliminated, it is possible to identify and remove bias from AI by taking steps to address concerns around fairness and bias. We can design AI in such a way that it meets our specific requirements, and a movement among AI practitioners, like The Future of Life Institute and Open AI, is already underway with the development of ethical and fair AI design principles. One of the key principles is that AI should be created in such a way that it can be audited and any bias can be located and eliminated. And if the tech doesn’t meet the specified standards, it should be improved before it can go into production.
Ethical considerations and dangers
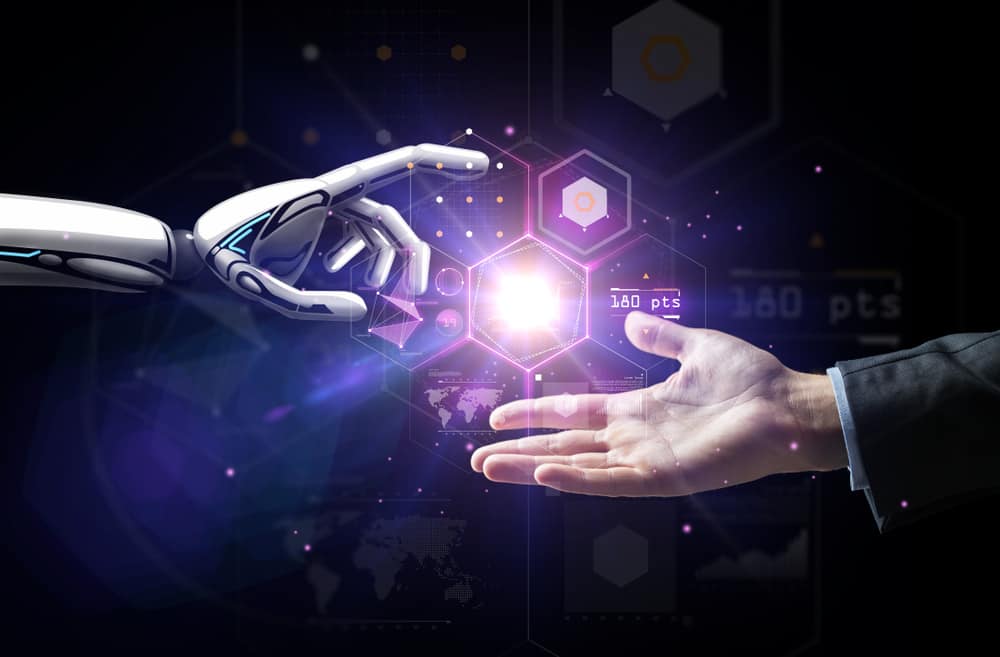
Artificial intelligence is becoming increasingly essential – not only in our daily lives, but also across a wide range of industries, including healthcare, retail, manufacturing, and even government. This technology does, however, also present some major challenges and ethical concerns. Think bias, discrimination, increasing surveillance, fake news, accountability, privacy, and more.
Privacy and consent
We might assume that all the data that is harvested by companies and organisations comes from adults who have given consent and are capable of making informed decisions around the use of their (private) information. But unfortunately, this is often not the case at all. Many companies that collect data sell it off to third parties, making it virtually impossible to keep track of what happens to your information. To make matters worse, much of the most privacy-sensitive data analysis tools – such as recommendation engines, search algorithms, and adtech networks – are driven by algorithmic decisions and machine learning. And as AI evolves, so does its ability to use private information in ways that are increasingly incomprehensible and intrusive.
Fake news
Neural networks that can create fake but hyper-realistic photo or video footage or flawlessly replicate someone’s voice are already widely in circulation on the internet. And while there are lots of opportunities to use deepfakes for good – such as in sectors like news and entertainment or education – they can also lead to significant threats, including the spread of misinformation, increasing criminal identity fraud, and even political tension. Deepfakes can mess around with agency and identity; they can be used to create videos in which someone does things he or she never did. And once a video is online, you lose all control over how the content is interpreted or used.
Discrimination and bias
Another growing concern is that machine learning systems can codify human bias and societal inequities reflected in their training data. There are already multiple examples of how a lack of variety in the data used to train such systems has negative real-world consequences. For instance, in 2018 an MIT and Microsoft research paper found that facial recognition systems sold by major tech companies suffered from error rates that were significantly higher when identifying people with darker skin – an issue attributed to training datasets being composed mainly of white men. And there are various other such studies. Another example of insufficiently varied training data skewing outcomes made headlines in 2018 when Amazon discontinued the use of a machine-learning recruitment tool that identified male applicants as preferable.
Accountability
Picture a medical facility using an artificial intelligent system to diagnose cancer and giving a patient a false-positive diagnosis. Or a criminal risk assessment system causing an innocent person to go to prison. Who – or what – will have to be held accountable for these grave mistakes? When an autonomous Tesla drove into a random pedestrian during a test drive, Tesla was blamed; not the human test driver sitting inside, and certainly not the algorithm itself. But what if the technology was created by dozens of different people and was also modified by the client? Can we then still hold the developer accountable? And in the case of medical or judicial errors where artificial intelligence is involved, who or what will be responsible?
AI is a black box
Artificial intelligence and machine learning models can significantly impact our lives. They are increasingly used to tell us which job candidate to hire or to help determine who is guilty of a crime and needs to be sent to jail. They decide which military target to bomb, or whether that spot on your chest is cancerous. As accurate as this technology can be, the problem is that we can’t always explain how it arrives at decisions. Tracing through the immense web of algorithmic decisions in order to figure out how these decisions were made is virtually impossible – and this poses problems. Imagine not being able to substantiate why one accused receives a two-year sentence for a crime, while another gets only three months for exactly the same crime. AI doesn’t explicitly share how and why it reaches its conclusions, and often all we know is that “the algorithm has decided”. And until we can get rid of these layers of obfuscation, some level of discomfort when it comes to putting our trust in this technology will remain.
AI in the wrong hands
When it comes to the true potential of artificial intelligence, we’ve only just begun to scratch the surface. It helps us expand our knowledge of human genetics, combat fraud and cybercrime, deliver breakthroughs in medicine, enable autonomous robots and vehicles, and so on. But regardless of the noble intentions we might have for the use of technology, there will always be people who will attempt to exploit it for personal gain, and artificial intelligence is no exception. Hackers are already using it to develop sophisticated phishing attacks and to arm vicious cyber offensives against unsuspecting targets. The malicious use of AI can lead to untold chaos in a myriad of ways; think faking data, stealing passwords, sabotaging computer systems or critical infrastructure, and more.
Dr Peter Stone of the University of Texas Austin says: “If someone today were to change all traffic signals in a city to be simultaneously green, disaster would ensue. And the fact that our electricity grid is fairly centralised makes us vulnerable to large-scale blackouts. The proper response would be stronger security measures, as well as redundancy – the provision of backup capacity – and decentralisation of decision-making.”
Will AI steal our jobs?
The future of work has arrived, and according to a report by the World Economic Forum (WEF), robots, automation, and artificial intelligence could replace 85 million jobs globally by 2025. But as job markets and the economy evolve, 97 million new roles will emerge in technology industries, in content creation fields, and across the care economy. Competencies where humans will likely retain their comparative advantage include advising, communicating, managing, reasoning, interacting, and decision making. Expectations are that there will be an increased demand for roles in areas like product development, cloud computing, and engineering, as well as in the data and artificial intelligence fields and in the green and blue economies. What is certain is that AI will change the nature of work, which leaves us with the question of to what degree and how rapidly automation will transform the workplace.
In closing
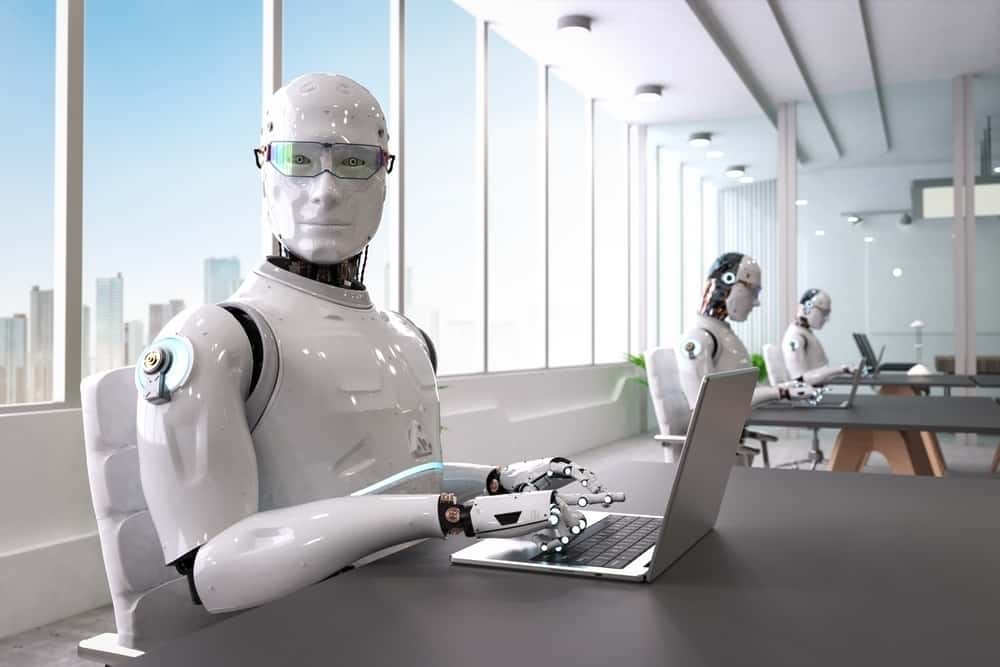
There isn’t an aspect of our lives that artificial intelligence doesn’t have the potential to impact. The technology is already used to recommend what you should watch, listen to, or buy next, to understand what you say to virtual assistants like Siri or Alexa, to recognise who and what is in a photo, or to detect credit card fraud.
While AI offers endless opportunities for improvement and innovation, it will not be able to achieve its full potential on its own. So, instead of fearing technology as something we need to compete with, our future with artificial intelligence will likely be about human-machine collaboration, and engineers, programmers, and everyday workers and consumers increasingly integrating AI into their daily lives. If we can combine mechanised precision and speed with human interaction, curiosity, and intuition, the possibilities will be limitless and we will be able to deliver unparallelled outcomes. Artificial intelligence unquestionably has the potential to greatly benefit humanity, provided we use it properly. We will, however, need to increase awareness around its limitations and challenges, and find ways to ensure that this technology is used responsibly and safely, and always for the benefit of all.