- We use them all the time, but what exactly are algorithms?
- When algorithms are used for good
- The horrifying consequences of algorithms gone wrong
- What can we do to make things better?
- The ‘computer-says-no’ dilemma
- A final observation
Algorithms are a major part of our everyday lives. Most of the time, we aren’t even aware that we use them, nor why. But the reality is that we couldn’t even function without them anymore. The internet runs on algorithms. Emails reach their destination because of algorithms. All our online searches are accomplished via algorithms and our smartphone apps wouldn’t work without them. And although algorithms have been created with good intentions – to improve our lives – they are increasingly causing major issues. They make mistakes, are biased, and can be used for criminal purposes. And to make matters worse, there are no regulatory or supervisory bodies (to speak of) to protect us from algorithms going wrong – yet. To add insult to injury, directors and decision makers don’t always have the right knowledge to base their decisions on – and civil servants and employees often leave decisions to algorithms that don’t function as they should. Proper supervision, and therefore protection, is lacking. Are we at the mercy of the ‘gods’?
We use them all the time, but what exactly are algorithms?
An algorithm is basically a set of rules or steps that can be implemented in various ways in order to achieve a certain objective. The steps in a recipe that you follow in order to create a meal, for instance, are an algorithm. And computer algorithms are the invisible mechanisms that determine, for instance, the recommendations we see on social media, Netflix, or on a fashion website. Algorithms solve problems. One example is the apps on our smartphones that help us find the most efficient route to a destination, and sometimes they also need to connect with other databases to gather real-time traffic information. Algorithms can predict the weather and help us with our next move on the stock market. They can help discover illnesses such as breast cancer, or identify fake news. They make sure our smart devices respond to our voice commands and recognise our fingerprints or our faces. They are used to track our every move and monitor which articles we prefer reading. They extract important data from these actions, so that they can offer reading suggestions. Algorithms can even be ‘instructed’ to discourage or prevent us from seeing certain types of information.
When algorithms are used for good
As mentioned before, computer algorithms have been created to assist us with a myriad of tasks and are intended to be used for good. They enable incredible levels of speed and efficiency, and lead to increased creativity and enhanced self expression. They help us crunch databases and can extract knowledge much faster than humans will ever be able to, and make decision-making, purchasing, transportation, and all kinds of other important tasks more efficient. In short, “If every algorithm suddenly stopped working, it would be the end of the world as we know it”, says trendwatcher and futurist Richard van Hooijdonk.
Algorithms for vaccine development
It used to take up to decades to develop a new vaccine. These days, however, vaccines are being developed at record speeds, and COVID-19 vaccines were already undergoing human trials a mere three months after COVID was first reported. These advances in vaccine development can partly be attributed to the algorithms that helped researchers analyse vast quantities of information about the coronavirus. Machine learning algorithms are able to sort through thousands of sub-components of virus proteins with lightning speed and predict which components are most capable of generating an immune response. This helps researchers design specifically targeted vaccines much faster than has previously been possible. This is a very promising development in the more than 200-year history of immunisation and may revolutionise the way vaccines are created, saving many lives in potential future epidemics. “AI is a powerful catalyst. It enables scientists to draw insights by combining data from multiple experimental and real-world sources. These data sets are often so messy and challenging that scientists historically haven’t even attempted these sorts of analyses”, says Suchi Saria, professor at the Johns Hopkins Whiting School of Engineering.
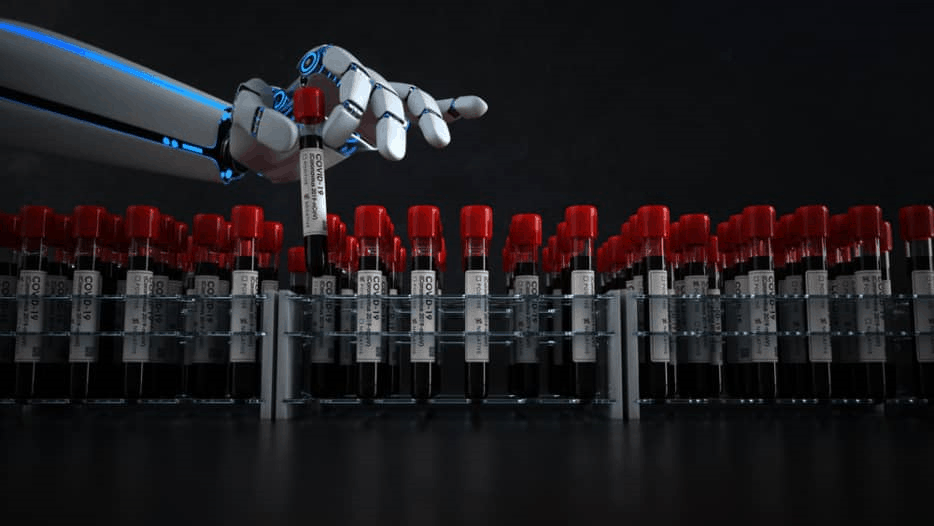
Algorithms for solving crimes
There are mountains and mountains of data to sift through in order to get to evidence that can be used to solve crimes. To make sense of all this information, and to discover patterns, we need a bit of help; from algorithms, for instance. Investigative psychologist Daan Sutmuller explains: “There’s a wide range of murders, while the features of a crime scene can overlap. For example, stab wounds can point to both a relational murder and a psychotic perpetrator. Because every murder case is unique, it has its own algorithm”. Sutmuller wants to develop a software package that contains a library in which the elements of evidence are assessed. “This will allow an analyst to build an algorithm for a particular case. By using the building blocks from the library and adapting them to the particulars of the case, the algorithm should reveal the persons of interest. The results should point the detective in a certain direction. This may require searching the victim’s social network or investigating the people who used their phone near the crime scene. The algorithm should support the detectives in making their choices.”
Algorithms to help you keep your customers
Companies can also use machine learning algorithms to help them retain their customers. Algorithms can analyse the signs that point to potential customer churn – the phenomenon where customers no longer purchase goods or services or interact with the company. By creating ‘churn models’, algorithms can predict the percentage of customers who are not likely to make a future purchase by observing and analysing which behaviour patterns or characteristics are predictive of a customer leaving. Churn models can be used to help companies decide on new marketing campaigns, product or service improvements, or whether to increase or decrease prices. Companies that are in constant competition to retain existing (and acquire new) customers will greatly benefit from applying churn models. Jack Welch, former chairman and CEO of General Electric, famously said: “There are only two sources of competitive advantage, the ability to learn more about our customers faster than the competition and the ability to turn that learning into action faster than the competition.” Now, we have algorithms for that.
Algorithms are designing better buildings
Algorithms can also give architects and engineers new tools to help them decide on the best way to design and construct buildings. Algorithms can assist architects by revealing patterns in proposed constructions, enabling them to work out how to lay out the rooms and get a better understanding of how space can be used in the most efficient way. Algorithms also offer solutions in terms of structural, spatial, and energy efficiency. Zaha Hadid Architects use algorithms for the automated testing of thousands of layout options and to find ways to create irregularly shaped buildings without them becoming incredibly costly. Algorithms have become increasingly important in the design of novel buildings, continuously adapting as they respond to environmental, structural, and usage data. Algorithms are even creating office layouts that enable the maximum number of people to be present while still being able to adhere to social distancing measures. When we combine the three uses of algorithms – revealing patterns, managing complex information, and generating new spatial arrangements – future algorithmic design will really change our ability to improve the built environment.
Algorithms can ‘accident proof’ autonomous cars – up to a point
In terms of complete road safety, the computer vision systems, cameras, and image processing capabilities of autonomous cars still aren’t developed enough to be able to detect when a road traffic user points, nods, or smiles. Even signals like flashing lights are difficult to interpret by algorithms, as they can mean anything from ‘there’s something wrong with your car’, to ‘watch out’, or ‘go ahead’ – depending on the traffic situation. Algorithms can, for now, make autonomous vehicles somewhat accident-proof, but only when other road users act responsibly and follow the rules of the road. The current algorithms can take unexpected situations into account, such as pedestrians or cyclists suddenly appearing. But humans are still much better at appropriately responding to unique situations, and only once autonomous cars can be trusted to drive more safely than human drivers, and respond to unique traffic situations, can they be widely adopted. We could give autonomous cars their own lanes, and over time, we could gradually increase these self-driving lanes until all vehicles are autonomous. Think of it as a train or tram system, but without the rails. The safest and easiest way to make autonomous cars a reality is to keep other road users out of their way.
The horrifying consequences of algorithms gone wrong
In many instances, it’s perfectly fine for AI to make low-risk decisions autonomously, such as serving the correct banner ad for the audience in question or providing the most efficient route home. But when it comes to more delicate matters or high-risk situations, such as correctly diagnosing a life-threatening illness, it becomes quite a different matter. Sometimes the workings of algorithms are so complex that even their creators struggle to understand them, which makes it difficult to completely trust what they are doing or figure out when they’ve made a mistake – until it’s too late. And while their potential for good is enormous, their potential for abuse might be even greater. When they are trained with wrong, incomplete or biased data or fall into the wrong hands, the consequences can be disastrous. We need to take into consideration that companies’ first goal is to maximise their profits, often even by repackaging profit-seeking as a societal good. We also need to be aware that we’re in the middle of an era where our privacy is becoming non-existent and manipulation in marketing is at an all-time high. Algorithms have the ability to read our minds and shape our thoughts and decisions – without us even being aware of it. Either this is the case, or we have become so used to relinquishing our privacy that we slowly but surely stop seeing this as a problem, especially when we get so much in return, such as increased comfort, personalisation, and efficiency.
Another problem is that algorithms are completely opaque, like an impenetrable black box. And to add insult to injury, it’s virtually impossible to determine accountability in terms of processes and decisions that are algorithm-based. Who do we hold accountable when a self-driving car causes a collision with another vehicle, or worse, runs over a pedestrian? Algorithmic decision-making can result in perpetual injustices toward the minority classes it creates, and will keep reproducing inequality for the benefit of a small, privileged part of the population that dominates the economy. Algorithmic decisions made for large corporations will eventually lead to the end of local entrepreneurship, local skills, local intelligence, and even minority languages. As Andrew Nachison, founder at We Media says: “The dark sides of the ‘optimised’ culture will be profound, obscure and difficult to regulate – including pervasive surveillance of individuals and predictive analytics that will do some people great harm (‘Sorry, you’re pre-disqualified from a loan.’ ‘Sorry, we’re unable to sell you a train ticket at this time.’). Advances in computing, tracking, and embedded technology will herald a quantified culture that will be ever more efficient, magical, and terrifying.”
Algorithms can fire you without any supervisor involvement
Amazon’s algorithm-based staff productivity tracking and termination processes automatically generate warnings – and even terminations – related to quality or productivity without input from supervisors, although supervisors do have the ability to override these decisions, according to Amazon. The problem with this is that algorithms don’t see people, they only see numbers. Amazon staff have repeatedly reported that they are being treated like robots that are monitored by automated systems. The systems even generate automated warnings when a worker’s break in between scanning parcels takes ‘too long’. A couple of these warnings can automatically lead to termination of employment. Some employees have even mentioned avoiding going to the toilet so that the system doesn’t flag those as ‘unnecessary breaks’ and get them into trouble. An attorney representing Amazon said the company fired hundreds of employees for inefficiency and unmet productivity quotas at a single facility between August 2017 and September 2018.
Algorithms can cause bias and increase inequality
There are numerous examples of algorithms leading to inequality. One such example is the US state of Indiana, where an algorithm unfairly categorised incomplete paperwork for welfare application as ‘failure to cooperate’. This resulted in millions of people being denied access to cash benefits, healthcare, and food stamps for three years. This also led to the death of cancer patient Omega Young, who was unable to pay for her treatment. The problem is that biased data fed into systems by biased people leads to biased algorithms, which in turn leads to biased outcomes and inequality. When we repeat past practices, not only do algorithms automate the status quo and perpetrate bias and injustice, but they also amplify the biases and injustices of our society.
In February 2020, the Dutch Court ruled that the SyRI (System Risk Indicator) could no longer be used in low-income areas to flag people with a higher likelihood of committing benefits fraud. The Dutch Ministry of Social Affairs first implemented the algorithmic program in 2014 to gather information about people living in low-income and immigrant neighbourhoods in various Dutch cities. The information was fed into predictive algorithms used to indicate the level or risk to benefits agencies. The court determined that by treating anyone living in the wrong area as more likely to commit a crime, SyRI constitutes a human rights violation with far-reaching implications for how human rights and privacy laws are applied to predictive algorithms. On 15th January 2021, the Dutch cabinet decided to step down over an escalating scandal in which tax officials wrongly accused thousands of parents of childcare benefits fraud, plunging many families into debt by ordering them to repay benefits. Dutch prime minister Mark Rutte said during a press conference: “Mistakes were made at every level of the state, with the result that terrible injustice was done to thousands of parents.” The above illustrates how algorithms can potentially be used for discriminatory purposes, and instead of technology becoming an equaliser, it often leads to the reinforcement of existing imbalances, bias and inequality.
Algorithms can wrongly predict healthcare needs and risks
Algorithms are also widely used to predict patients’ future medical requirements. In the US, for instance, risk prediction algorithms are applied to millions of patients to determine who would benefit from extra medical care now, based on how much they are likely to cost the healthcare system in the future. These predictive machine learning algorithms become more accurate as they are fed new data, but it was discovered that they have unintended consequences. For instance, according to health researcher Ziad Obermeyer, “black patients who had more chronic illnesses than white patients were not flagged as needing extra care.” This was caused by the fact that healthcare costs were used as a proxy for illness, leading the algorithm to make predictions that were only applicable to white people, for whom fewer health conditions led to lower healthcare costs. But for black Americans, the reasons for lower healthcare spending are different – such as lack of insurance, inadequate healthcare, or other barriers to healthcare access.
Algorithms can wrongly accuse and convict the innocent
US courtrooms, under enormous pressure to reduce incarcerations without risking increasing crime rates, have turned to algorithms to get people convicted as safely and efficiently as possible. Law enforcement agencies increasingly make use of facial recognition technology to identify suspects, but do these systems improve crime stats or actually perpetuate existing inequalities? According to civil rights advocates and researchers, “facial recognition tech can fail spectacularly, particularly for dark-skinned individuals – even mistaking members of Congress for convicted criminals.” Things get really bad, however, when it comes to criminal risk assessment. The algorithms developed for this only carry out one task, which is using the details of a defendant’s profile to generate a recidivism score to indicate the likelihood of reoffense. The score becomes part of a host of decisions to determine the severity of the sentence. The problem is that minority and low-income groups – communities that have previously been targeted disproportionately by law enforcement – are at risk of getting high recidivism scores. This leads to perpetuated and amplified bias, which generates even more biased data feeding an increasingly biased vicious cycle. And to make the situation even more complicated, the algorithms are proprietary and opaque, making it virtually impossible to call their decisions into question. While civil rights organisations continue to debate and try to halt the use of these tools, more and more jurisdictions and states are actually implementing them in a desperate attempt to fix the mess of their overburdened correctional facilities.
Deepfake algorithms make it impossible to know what’s real and what isn’t
When we hear about deepfakes, we tend to think of sites like Deepnudes, where any face can be superimposed on (pornographic) video content, as a potential worst case scenario. But when you consider that many nations are currently actively involved in cyberwars, things can actually turn out much, much worse. Initially created for entertainment, deepfakes can be used – and have already been used – as dangerous weapons in the war against truth. The technology makes it very easy to create hyper-realistic video content depicting events that, in reality, never took place. Citizens, law enforcement officials, and legislators are increasingly concerned about deepfakes’ potential to target individual people, incite violence, and even interfere with our democratic discourse and election results. And since this technology is evolving at incredible speed, it’s becoming more and more complicated to distinguish deepfakes from authentic video content. In a world where we have long embraced the belief that ‘seeing is believing’, this technology poses a significant threat. A well-scripted and perfectly-timed deepfake or series of deepfakes could sway elections, spark violence, or exacerbate political divisions in a society. We need to urgently find a solution that takes into consideration the fact that the technology and the way it is used will continue to evolve. Until then, we will have to accept that seeing isn’t always believing.
What can we do to make things better?
The development of algorithms has been intended for the purpose of optimising everything in our lives – make things easier, make sense of chaos, and even save lives. Many experts are, however, of the opinion that they put too much control in the hands of governments and corporations. They create filter bubbles, limit our choices and our creativity, lead to and perpetuate bias, and increase inequality and unemployment. We are already relying more and more on algorithms and machine learning. We do, however, need to ask ourselves how we can better manage and understand the situation we’ve created. Humans and technology seem to have reached some form of partnership where algorithms aren’t in control, but where they are created and adjusted by people. Effects that are positive for one person can, however, be negative for another. And because tracing cause and effect is complicated, it’s important to keep adjusting these balances and try to understand how algorithms work. If we look at the enormous potential of the use of technology, it’s likely that a general trend toward positive outcomes will prevail.
To make sure algorithms keep improving our lives and to try and keep the downsides of this tech to a minimum, it’s important for all of us to get a better understanding of their workings, of what the potential pitfalls are, and how the use of algorithms can affect people’s lives. We also need to gain a thorough understanding of the ethical implications of algorithmic systems, and behavioural science – which encompasses philosophy, anthropology, sociology, and psychology – can help provide a comprehensive lens.
Hippocratic oath for data scientists, statisticians, and mathematicians
Some out-of-the-box thinking could also help us minimise ‘algorithms going wrong’. We could, for instance, implement an ethical pledge for data scientists and mathematicians, a kind of Hippocratic oath, just like medical doctors. This pledge could be something along the lines of “I swear by Hypatia, by Lovelace, by Turing, by Fisher (and/or Bayes), and by all the statisticians and data scientists, making them my witnesses, that I will carry out, according to my ability and judgement, this oath and this indenture.” Although such a pledge may have assisted the developers of some of these problematic algorithms, bias awareness training could have made a huge difference as well. What also comes to mind is ‘privacy-by-design’, ‘bias-by-design’, and ‘ethics-by-design’ – data processing procedures already integrated in algorithms when they are created.
If there were ever to be an ethical pledge for the development of algorithms, it should also contain a code of standards. For instance, details on the data with which an algorithm is trained should be made available, and each algorithm should come with an explanation of its function and objectives, and be open to auditors for testing various impacts. The public sector should also regularly evaluate the impact of algorithms used in decision making processes and publish the results. And perhaps most importantly, citizens should be made aware when decisions that impact their lives were based on or informed by an algorithm, whether fully or partially.
List of principles to guide and inform the ethical use of AI algorithms
It’s high time we ask questions about the ethics of the algorithms used by organisations, governments, and businesses. More and more tech companies are now formulating principles to guide them. Facebook, Google, IBM, and Microsoft, for instance, have developed the Partnership on AI, a framework dedicated to the use of AI for social good and to address the ethical issues related to artificial intelligence. DeepMind has started a research initiative on Ethics and Society to assist companies in ensuring that AI is held to the highest ethical standards, encompassing human rights, welfare, justice, and virtue. Some examples of principles regarding the use of algorithms include:
- Algorithms used by organisations should be accompanied by a description of their function, objectives, and intended as well as potential impact. This description should also be made available to its users.
- The details describing the data with which an algorithm was and still is trained – and the assumptions used, including a risk assessment for dealing with potential biases – should be published.
- Organisations will need to adhere to internationally recognised principles of human rights. Their algorithms should conform to the right to property, privacy, religion, freedom of thought, life itself, and due process before the law.
- Organisations should aim to create and use algorithms that provide the greatest possible benefit to people all over the world – algorithms that increase human welfare by improving healthcare, education, workplace opportunities, and so on.
- Algorithms should come with a ‘sandbox version’, so that the impact of various input conditions can be tested by auditors.
- Organisations need to commit to evaluating the impact of the algorithms used in decision-making processes and publish the results.
- People need to be informed about whether their treatment or any decisions that affect their well-being were informed by, or based on, algorithms.
- Organisations should aim to achieve social justice in their development and use of algorithms and avoid algorithms that proportionally disadvantage certain groups of people.
- Organisations should design and use algorithms that contribute to human flourishing and enable affected people to develop and maintain character traits like honesty, empathy, humility, courage, and civility.
Algorithm watchdogs and audits
Algorithms are used to inform all kinds of decisions – from applications for benefits to building inspections and more – but in some cases, they have proven to be biased against certain gender, class and racial groups. What’s more, some of these algorithms are so complex that even their creators often can’t figure out why they make certain decisions or recommendations. This is not only true for algorithms used in the public sector, but also for those used in business. At the present moment, the algorithms used online are subject to little or no regulatory oversight and increased monitoring is required by regulators. It is critical to have algorithm auditors who can regulate algorithm decision-making processes so that our privacy can be safeguarded and that we are protected from discrimination, bias, and any other potentially adverse consequences. These special auditors should have a solid knowledge of ethics and a thorough understanding of how algorithms impact our lives. They should ensure that algorithms can be explained, and that they are ethical and transparent. Fortunately, we are beginning to see coalitions form between lawyers, activists, researchers, concerned tech staff, and civil society organisations to support the accountability, oversight, and ongoing monitoring of AI systems.
Mitigating algorithmic bias
It can be safely asserted that if a data set is complete and comprehensive, bias can really only creep in as a result of the prejudices of the people working with these data sets. Fully removing bias is, however, quite a bit more complex than it sounds. One could, for instance, remove race, sex, or other labels from data but that would affect the accuracy of the results and diminish the understanding of the model. Here’s a few recommendations on how to mitigate algorithmic bias, based on expert opinions:
- Diversify your teams – As algorithms can really only be as inclusive as the people who develop them, the most obvious solution is to make sure you have lots of diversity in your teams. It’s become very obvious that there’s a painful lack of diversity in the tech industry – which is where algorithms are developed. According to the findings of a study accepted by the Navigating Broader Impacts of AI Research at the 2020 NeurIPS machine learning conference, the more homogeneous a team is, the more likely it is that a given prediction error will appear twice. More diverse teams will reduce the chance for compounding biases.
- Use the right data and information to create your algorithms – The caustic observation ‘garbage in, garbage out’ carries a special warning for machine learning algorithms. How can we expect an algorithm that’s based on a small subset of the population to work for all of us? We need to be aware that bad data can rear its ugly head twice – first in the historical data used to train an algorithm, and second in the new data used to make future decisions. Complex problems not only require more but also more diverse, and more comprehensive data in order to produce comprehensive models. We need to keep in mind that one minor error during one step will cascade and cause more errors across all subsequent steps in the entire process.
Factoring in a layer of humility
Algorithms learn and reach decisions in increments as they progress. First, they learn through inductive learning, or learning patterns through groups of data. Then, they start making predictions through deductive learning, for instance ‘given these inputs it will always be this output’. When in production, they use abductive learning – for instance, the logic that all dogs have fur. According to algorithm logic, any given animal that has fur should, therefore, be a dog. It’s not hard to see how this type of learning – if left unmanaged – can cause problems. ‘Humble AI’ tries to prevent this. If we can teach an algorithm to steer clear of these logic leaps, perhaps we can then also trust it to check its own reasoning and make sure it makes sense in real life situations. Algorithms can then also eventually prevent faulty or biased reasoning from becoming part of their decision-making stages.
Consider whether automation is appropriate or necessary
The algorithms that we, as humans, execute are much more flexible than the rigid and increasingly ubiquitous computer algorithms dominating our everyday lives. Even flexible automation is considerably less flexible than humans. Unlike computers, humans can make a decision within a split second – we are, in fact, the most versatile machines of all. We also need to take into account that automation technology could eventually subjugate humankind instead of serve it, with ever dwindling privacy and loss of freedom as a result. Human error in the management of artificial intelligence could also increasingly endanger society itself, with humans becoming more and more dependent on automation for their (economic) well-being. It is, therefore, critical to determine whether or not it is appropriate or even necessary to automate.
The ‘computer-says-no’ dilemma
Richard van Hooijdonk, renowned trendwatcher and futurist, says: “It’s important for administrators and decision-makers to be aware of the consequences of their decisions. From conversations I had with government officials and some MPs it became clear that decision-making by algorithms was not based on their own knowledge and insights. This is problematic. An understanding of how technology works, and being able to link the risks and social effects to it, is of critical importance for proper assessment and decision-making. We owe this to our citizens and consumers. Algorithms also seem to be the perfect excuse within the operational corporate and government apparatus for people to not have to think for themselves. The ‘computer-says-no’ effect is all too common now, but thanks to increasingly smarter, self-thinking algorithms, civil servants and employees will soon have even less reason to oppose a decision or recommendation spat out by an algorithm. Critical thinking and human intervention are therefore crucial – certainly in the current landscape, in which we are still only experimenting with this new technology. I am not generally in favour of the ‘surveillance economy’, but in this particular case I am very much in favour. Supervision is important in the pursuit of justice and in this case it also offers us learning opportunities. The current organisations that exercise this supervision, such as privacy authorities, have insufficient knowledge and do not have the right culture to conduct this type of supervision. Digital supervision requires new knowledge about new developments (technology, ethics) and must anticipate potential situations that we have never experienced before. It’s therefore critical to urgently set up more agile digital supervisory bodies. Consideration should also be given to vertical digital surveillance. Algorithms are used in all sectors, but predominantly in healthcare, logistics, and retail. And they all require a different type of application of technology and ethics and therefore require a different type of supervision and enforcement. So, at each sector level (‘vertical’), specialists will have to work towards a safer and fairer society.”
A final observation
Rapid developments in artificial intelligence offer opportunities as well as dangers, and fundamentally change the way we live. Algorithms can make the world of work – and our lives – more efficient, safer, and more comfortable. They can, however, also be used to create fake content that is indistinguishable from the truth, decide on who is and isn’t ‘worthy’ of government benefits, and determine who is a potential reoffender. What’s more, in many cases, as algorithms become increasingly complex, humans no longer fully understand how they arrive at their decisions. And because most are opaque, it’s virtually impossible to investigate their workings. With algorithms becoming an increasingly critical part of modern existence, concerns about their potential malfunctioning have, thankfully, prompted initiatives towards the creation of unbiased, ethical, and transparent governance frameworks and principles. And even though not everyone might always agree with these frameworks, they will provide critical general guides to the development of ethical data practices and ensure that algorithms will, first and foremost, provide benefits to people all over the world and contribute to human flourishing.