- Safer, healthier environments
- Improved crime detection and personal safety
- AI in disaster management and prevention
- Increased safety on our roads
Technology is increasingly used to help improve security and keep us safe from harm. Advances in sensor technology, cameras, artificial intelligence (AI), facial recognition tech, and smart robotics have enabled us to develop improved systems that help us protect ourselves, our homes, public spaces, and the environment. Artificial intelligence in particular is becoming more and more essential to our wellbeing and is increasingly used to improve crime detection, prevent natural disasters, and increase the safety on our roads.
Safer, healthier environments
AI has the potential to improve and accelerate worldwide efforts to conserve resources and protect the environment by monitoring CO2 emissions, pollution, and deforestation. At this moment, various sustainability and environmental projects and initiatives are underway that use big data and artificial intelligence to tackle environmental challenges, monitor wildlife, and prevent forest fires, among other things. AI will be deployed to many more issues that threaten our environment and exacerbate climate change.
Our rainforests are the lungs of the world, providing us with oxygen, and a world without rainforests is virtually incomprehensible. Yet deforestation continues to clear these essential animal and plant habitats on a massive scale, posing a serious threat to all life on earth.
Using AI to preserve the forests of the world
Approximately 31 per cent of the global land area is covered by forests and is home to over 1.3 million animal species. Our rainforests are the lungs of the world, providing us with oxygen, and a world without rainforests is virtually incomprehensible. Yet deforestation continues to clear these essential animal and plant habitats on a massive scale, posing a serious threat to all life on earth. To protect our forests, researchers around the world are increasingly making use of artificial intelligence. Data science company Gramener, for instance, uses AI and machine learning in the fight against illegal deforestation and other natural disasters caused by humans. The company recently joined forces with a plantation company in Southeast Asia to ensure responsible harvesting by cutting down only those trees that have reached a certain level of maturity. To make deforestation economically as well as ecologically viable, the plantation company previously needed to manually analyse aerial footage to determine the age of its trees, ensuring they were mature enough and wouldn’t grow any further. Understandably, this process was very time consuming. To improve this process, Gramener developed an AI system that can automatically identify these mature trees from photographs. Anand Subramanian, CEO and cofounder of Gramener, says: “The system is now automated. This is not something that necessarily requires an expert, but the area of coverage is so large that automation makes a huge difference in this case.”
Unfortunately, lots of illegal logging still takes place. In fact, illegal logging accounts for anywhere between 50 and 90 per cent of all logging performed worldwide, says Topher White, renowned physicist, engineer and founder of the San Francisco-based nonprofit Rainforest Connection. White has developed an ingenious sound monitoring system called RFCx that analyses changes in forest sounds to detect signs of illegal logging activity, using repurposed cell phones placed high up in trees. These ‘RFCx guardians’ as they are called, notify authorities as soon as they detect unusual activity. The phones run on solar cells, enabling them to record sound 24 hours a day. The audio is then analysed with the help of AI and machine learning to identify the sounds of people, vehicles, chainsaws, logging trucks, and gunshots. The surveillance method has proven effective at stopping illegal logging in Latin America and central Africa. White said, “In terms of what you can do with the software – streaming it to the cloud, being able to analyse it up there – that’s when the really amazing stuff starts. They’re actually catching all the sound and launching it up to the cloud. We grab the data, we organise it, and then we can use it. Our networks actually pick out the sounds of chainsaws, logging trucks, animal species, all the rest.”
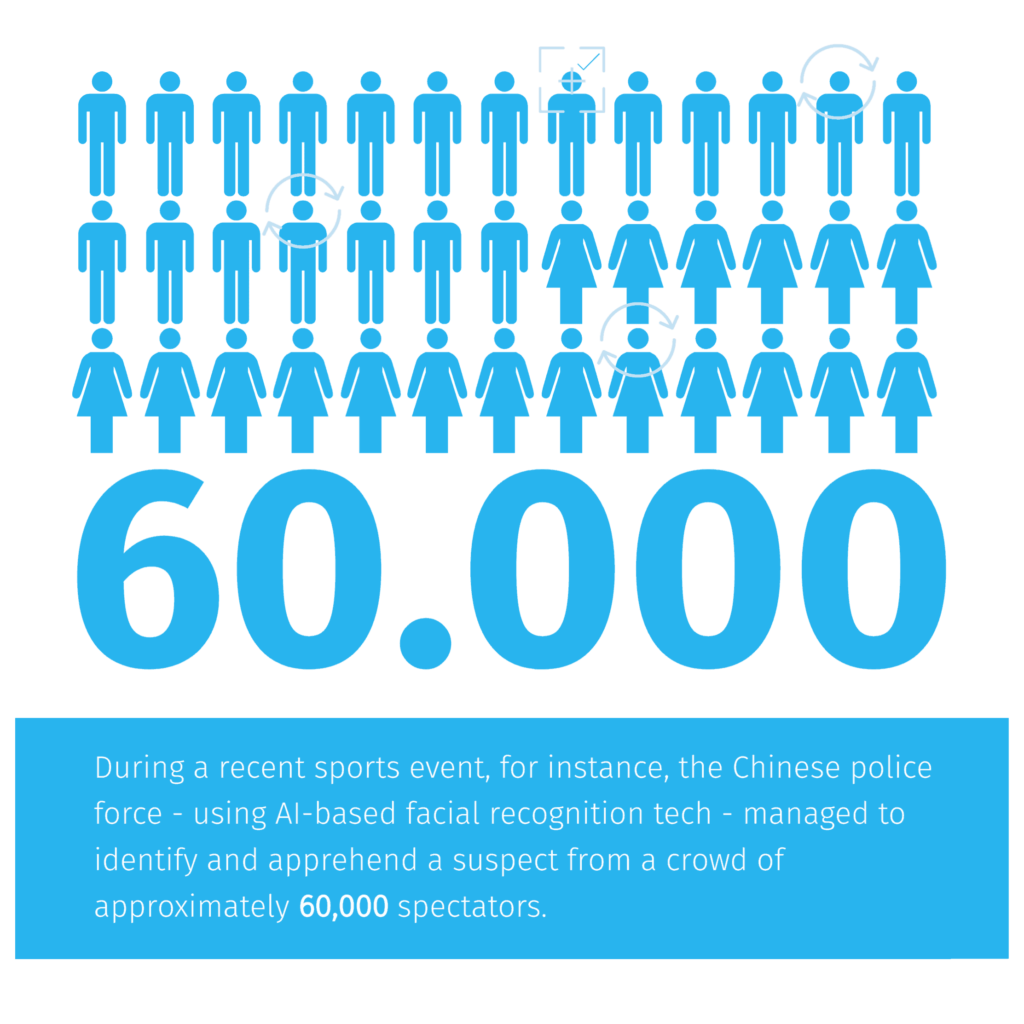
Protecting our oceans and preventing overfishing
AI, combined with satellite data and information about the movements of fishing vessels can be used to protect oceans from illegal overfishing. An example is Global Fishing Watch, a free intuitive and interactive online tool developed by Google, SkyTruth, and Oceana, that enables anyone in the world to monitor the activities of 35,000 of the world’s largest commercial fishing vessels in real time. The platform makes use of neural networks to enable the identification of areas where illegal fishing takes place, protecting marine life, preventing overfishing, and enabling the rebuilding of fish stocks and ocean habitats. Global Fishing Watch is a powerful tool and will have an immeasurable positive impact on ocean health. It will assist enforcement agencies in deterring illegal fishing, and enable commercial fisheries to better manage and understand their fishing activities. Jacqueline Savitz, vice president for Global Fishing Watch at Oceana, said: “Global Fishing Watch will revolutionise the way the world views commercial fishing. This free tool can be used by governments, journalists, citizens, researchers and the seafood industry. It will allow governments to track suspicious vessels, enforce rules and reduce seafood fraud. Global Fishing Watch is a powerful tool in the fight against illegal fishing and has tremendous potential to preserve and protect our world’s delicate marine ecosystem for generations to come.”
Improved crime detection and personal safety
The primary goal of law enforcers is to ensure that all people enjoy a safe and secure life by preventing and resolving crimes. Conventional crime fighting techniques are, however, no longer effective enough. They are slow-paced, labour-intensive, costly, inefficient, and often produce inaccurate results. AI is playing an increasingly important role in law enforcement and crime prevention. AI algorithms can identify, locate, and arrest real or potential criminals faster than ever, and prevent innocent people from being arrested. AI can be used to quickly and efficiently sift through huge amounts of data, images and video footage and other information, in order to detect patterns and make predictions. AI-based facial recognition technology is becoming increasingly widely used to identify an individual from a digital source like an image or a video. It can even be used to quickly identify a person in crowded areas in real time. During a recent sports event, for instance, the Chinese police force managed to identify and apprehend a suspect from a crowd of approximately 60,000 spectators.
Faster video analyses
AI enables much faster and more accurate video analysis. AI video analytics makes use of algorithms to differentiate between object types, identify certain behaviours or actions in real time, and provide alerts and insights. AI video analytics technology can automatically generate descriptions of what is happening in the video. This information can be used to track cars, people, and various objects detected in the footage, as well as their location and movements. Law enforcement officers can use this data to screen or finetune their search and look for specific characteristics – such as a tall man in a white shirt who walks at a certain speed, or a short woman carrying a red handbag – eliminating other results from the feed. Once a person has been identified as an individual of interest, the technology can then even locate that same individual on other video feeds and trace his or her movements.
AI-based audio recognition systems
AI-based audio recognition systems can help law enforcement officers identify a criminal through his voice by matching samples of speech from live conversations, phone calls, or footage on social media platforms like Facebook, Twitter, LinkedIn, Google+, and YouTube to those in databases shared by law enforcement agencies. Using search criteria like geolocation and language relevance, the systems can tag and process the voice recordings, and find similar footage in the databases. From audio samples – such as when a criminal says something during an incident or even ambient conversation – all the required information about the person can be accessed. Speech analysis algorithms can filter audio samples by age, language, gender, and even accent. These samples can be used to find a suspect’s name, date of birth, and so on, and provide information regarding recent involvement in criminal offences or previous arrests. One such platform is managed by Interpol and studied by security groups in the UK, Germany, Italy, and the Netherlands to evaluate its ethical aspects.
Predictive policing
AI can also help identify potential criminals so that law enforcement agents can question them when they suspect a criminal act is about to take place. Predictive policing can also identify specific areas where crimes are most likely to occur and even anticipate a specific date or time. This enables the deployment of police presence in certain areas at certain periods of the day or week to help prevent crimes from taking place. It also helps police departments with streamlining the allocation of policing resources. PredPol, for instance, a programme designed by UCLA scientists in collaboration with the Los Angeles Police department, makes use of big data and machine learning technology to anticipate the areas where a crime has the most probability to occur during a specified period. The software analyses data of past crimes to predict when and where the next incident is most likely to happen, leading to a significant reduction in crime.
Gunfire detection systems
Gunfire detection systems (GDS) can detect gunfire through AI-powered sensors. These sensors detect the gunshot sound while machine learning algorithms identify the place from where it originates and alert law enforcement officers. One such AI-based GDS system is ShotSpotters, which is already widely used across the US, providing evidence in increasing numbers of court cases. The ShotSpotter website reads that the system helps stop gun violence by using sensors, algorithms and artificial intelligence with which it classifies 14 million sounds in its proprietary database. ShotSpotter’s algorithms – which the company touts as virtually foolproof – are the company’s most valuable selling point. The workings of the system are closely guarded, however, and it is regarded as a largely inscrutable black box. What’s more, the methods used to identify gunshots do not always solely rely on the algorithms’ findings. In fact, employees are even able to alter the source of the sounds captured by the sensors, modify the number of shots, and alter the location, which introduces the potential of human bias into the algorithm and calls into question the legitimacy of these technologies.
Autonomous reporting, monitoring, and response systems
In the future, autonomous security systems will communicate with people and with each other, independently collect more information, and trigger complex security processes. Smart security technology will increasingly make use of remote monitoring capabilities and predictive intelligence and will be integrated in building systems. Autonomous, proactive, and real-time surveillance and response will be made possible by combining various technologies. Robots, for example, will provide assistance in the form of an extra pair of eyes that can gather up-close intelligence and inspect and evaluate a potentially dangerous situation – using sensors to check air quality, humidity, temperature and more – before dispatching humans to the site. Facial recognition and matching algorithms, combined with intelligent person search technology, can assist with the identification of suspects, help block access to unauthorised individuals attemtping to enter a building, or locate a person of interest
AI in disaster management, prediction, and prevention
From evacuation models to early warning systems – artificial intelligence, and machine learning in particular, enables a number of promising innovations for disaster management, prediction, and prevention. Think the forecasting of extreme (weather and climate-driven natural) events, the real-time detection of events, the development of hazard maps, and the provision of decision support and situational awareness. AI can be trained with information from seismic imaging to learn about earthquake patterns in order to be able to predict where and when an earthquake and aftershock might hit. When AI is trained with geographical and seismic data, it can learn to generate increasingly accurate forecasts of future volcanic eruptions. And when it comes to extreme weather conditions, AI is also increasingly used to monitor satellite footage to predict the force and course of tornadoes or hurricanes.
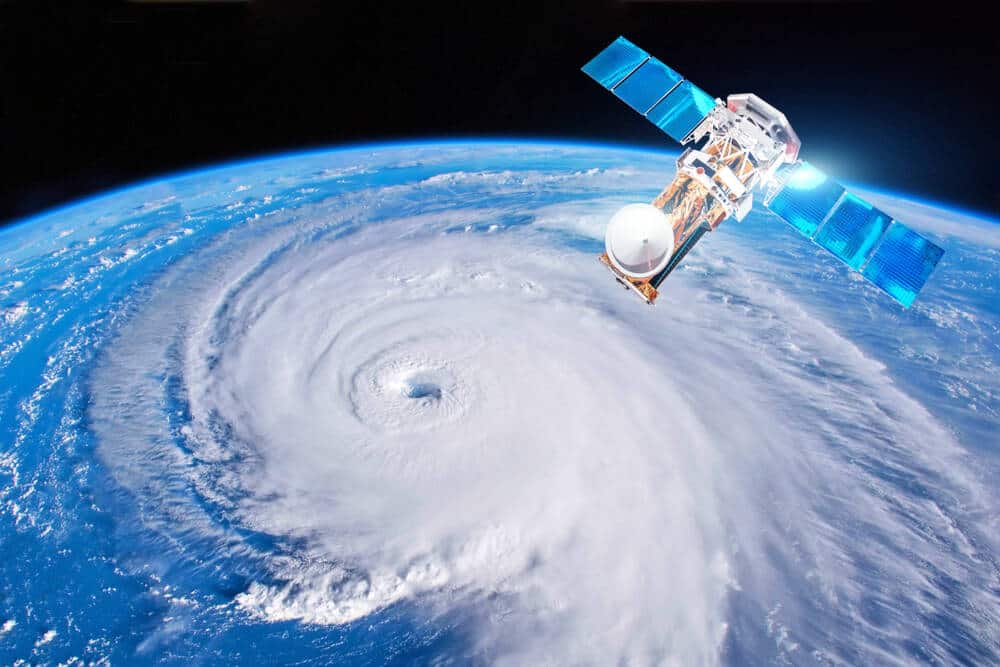
Prediction of severe weather conditions
In Georgia, the United Nations Development Programme (UNDP) is developing a multi-hazard early warning system to help mitigate the exposure of communities, infrastructures and livelihoods to severe climate and weather-driven events. Experts are making use of AI to develop a machine learning model that can help predict the probability of a severe weather event, such as wind- or hailstorms. The model can predict severe conditions by combining on-site observations with data from European Centre for Medium- Range Weather Forecasts’ 5th-generation atmospheric reanalysis dataset (ERA5) and National Oceanic and Atmospheric Administration’s 70-year Storms Events Database. The tool makes use of transfer learning techniques to extrapolate historical information from data-rich regions to locations all over the world that have limited data availability. Using ERA5 data and the Weather Research and Forecasting (WRF) numerical weather prediction model, extreme weather events can be simulated and analysed and used to predict severe storms and develop hazard maps.
DeepShake neural network predicts earthquake intensity
When it comes to earthquakes, even a couple of seconds of advance warning can make a difference in helping people prepare and take basic precautions. In fact, this can save tens of thousands of lives. Researchers at Stanford University have used deep learning algorithms to create DeepShake, a neural network that can quickly predict strong shaking and send out early warnings. DeepShake is trained with data from more than 35,000 earthquakes, and analyses seismic signals in real time. The neural network observes the earliest detectable waves from an earthquake, after which it is able to predict ground shaking intensity and send out alerts to the affected areas. DeepShake was developed with data from the Ridgecrest sequence of earthquakes in Southern California in 2019, and when tested, the neural network managed to send out simulated warnings to seismic stations in the area up to 13 seconds before high intensity shaking began.
Existing early warning systems are based on data from multiple sources, and they first determine the magnitude and location of an earthquake before calculating ground shaking intensity for a particular area. And according to Stanford student Daniel Wu, these steps can each introduce errors that can render a forecast less accurate. The DeepShake network, however, has learned which aspects of seismic waveform data most accurately predict the intensity of future shaking, and bases its early warning system solely on seismic waveforms. With only 15 seconds of measured ground shaking data – and without any prior knowledge of the location of seismic stations in its network – it is able to predict future shaking intensity at all of these stations. Wu explains: “DeepShake aims to improve on earthquake early warnings by making its shaking estimates directly from ground motion observations, cutting out some of the intermediate steps used by more traditional warning systems.” The researchers hope DeepShake will be able to fulfil a complementary role to the ShakeAlert warning system currently used in California.
As a result of road traffic accidents, approximately 1.3 million people lose their lives every year, and up to 50 million more are injured or suffer accident-related disabilities.
Source: World Health Organisation
Better safety on our roads
There are more and more vehicles on our roads today than ever before, leading to an increased risk of accidents and associated injuries and deaths. In fact, as a result of road traffic accidents, approximately 1.3 million people lose their lives every year, and up to 50 million more are injured or suffer accident-related disabilities, according to the World Health Organisation (WHO). Road traffic accidents are the leading cause of death for children and young adults and cost most countries 3 per cent of their GDP. AI can help improve road safety in different ways, including enhancing road infrastructure, better collection and analysis of crash data, and increasing the efficiency of post-crash responses. AI is already used to improve driving safety. Think connected vehicles that communicate with road infrastructure and each other, as well as mobile apps that monitor driving behaviour and reward responsible drivers with lower car insurance premiums and other perks.
Autonomous vehicles
Most car accidents involve some type of human error and are potentially avoidable. With the increasing implementation of 5G and AI, autonomous cars – using radar sensors and cameras to create a 3D view of their surroundings – will eventually be able to remove much of the human error out of split-second decision making, decreasing the number of collisions and improving safety on our roads. Improved connectivity will soon enable the capturing and transmission of real-time information to enable the analysis of collected data needed to make many of these important decisions. Autonomous vehicles can detect objects and pedestrian traffic and differentiate between people and inanimate objects. Today’s AI-based dashcams and sensors combine integrated technology that enables autonomous vehicles to detect pedestrian walkways a few kilometres ahead, providing drivers with the information needed to navigate pedestrian-heavy cities. Vehicles with integrated AI can mitigate human error by reminding drivers to follow traffic rules, drive in the correct lane, and maintain a safe distance from other road users.
Traffic management
In transportation, AI is used in combination with closed-circuit television cameras and sensors to record valuable information while on the roads, such as traffic and weather conditions, in order to warn and prepare drivers for adverse conditions and delays. The harvested data is stored in the cloud, enabling fast and efficient reporting and predictions on the status on our roads. AI enables traffic congestion predictions, providing authorities with relevant, actionable data and ample time to plan the allocation of resources in order to relieve bottlenecks, make travelling as hassle-free as possible, and avoid costly delays. This way, AI not only helps reduce urban congestion but leads to a reduction in CO2 emissions as well. Siemens Mobility recently developed an AI-based monitoring system that is used to process video feeds from traffic cameras. The system automatically detects irregularities, such as traffic congestion or accidents, and alerts traffic management authorities. The system can also estimate road traffic densities and then modulate traffic signals accordingly to improve traffic flow. The implementation of AI and machine learning will enable more and more intelligent transportation applications, which will improve traffic safety for all road users.
In closing
Research shows that artificial intelligence can tackle all kinds of complex and time consuming tasks better than humans can. And from the various examples in this article, we have seen that we can also harness AI to help us create a healthier, safer world. AI can help us mitigate human error, for instance while driving, and reduce the number of dangerous situations that lead to accidents. Artificial intelligence is also increasingly used to help us detect and prevent fraud, fight crime and combat illegal activities like deforestation and overfishing. AI may eventually even enable us to create a kind of digital dashboard for our planet with which we can monitor and manage our worldwide environmental systems, improving our sustainability efforts and enhancing our ability to predict natural disasters better. Artificial intelligence in all its forms is truly transformative technology that can help us create a world that’s safer for all.